When It Comes to Artificial Intelligence, ‘Big Data’ Isn’t Everything
While data are crucial to AI development, many other factors also contribute to companies’ competitiveness in the market
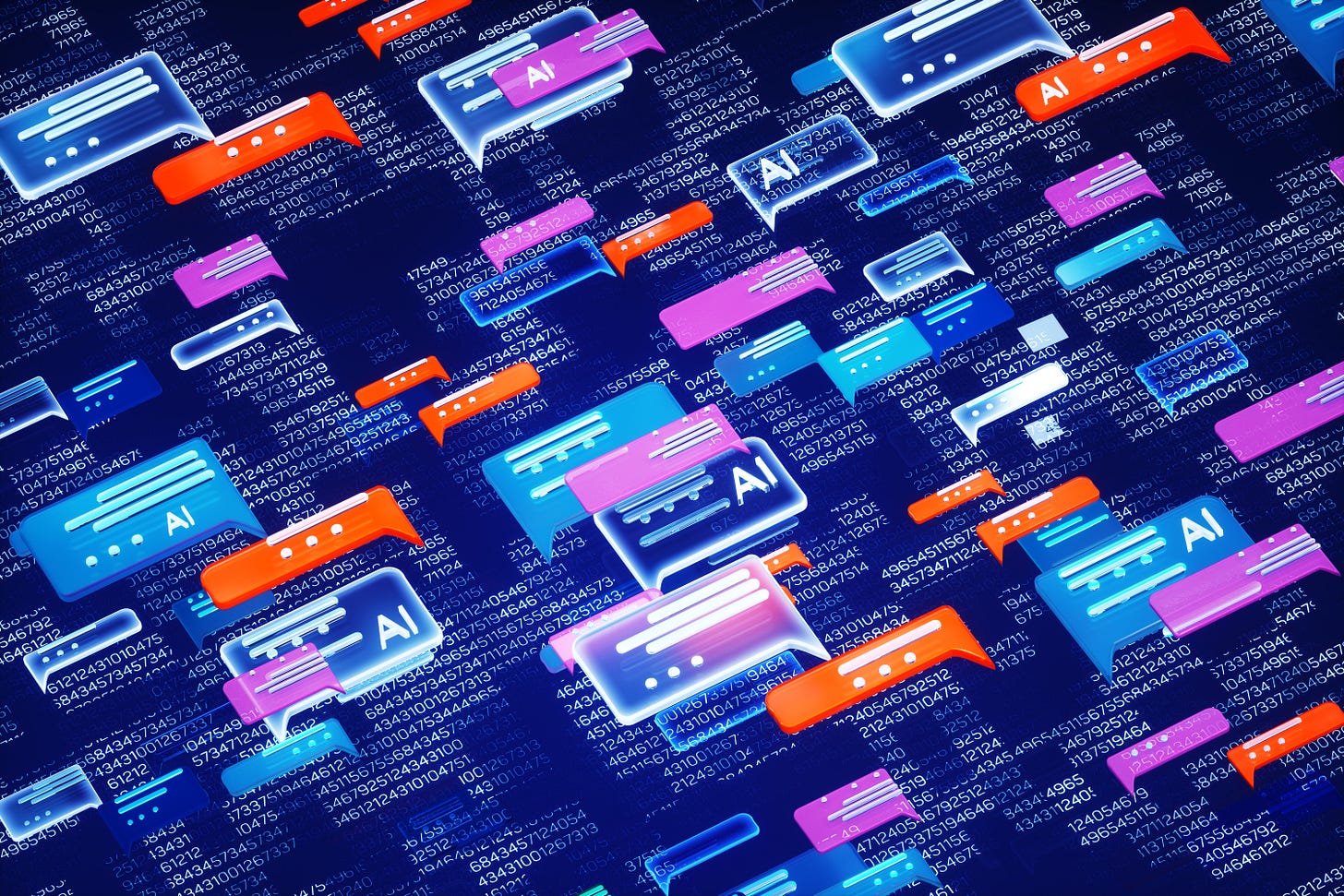
Seven years have passed since The Economist compared “big data” to oil and hailed data as the world’s most valuable commodity—and those claims seem to be truer now than ever. Today, rapidly evolving artificial intelligence (AI) technologies amass and assimilate troves of data to provide informed insights to businesses and governments on everything from consumer behavior to the evolution of disease-causing pathogens and the development of new medicines. Generative AI also facilitates the creation of art, images, sound and movies. These technologies and the predictive software foundation models on which they’re based wouldn’t be possible without data.
When an AI model aims to analyze, make predictions about or infer preferences from a population or consumer base, it’s easy to see how a larger dataset of individuals with more detailed information about them and their behavior could give some AI developers an advantage over their peers in producing better models and applications. As AI tech and models have rapidly evolved to make finer inferences and produce increasingly complex outputs, the number of parameters on which the model seeks inferences has expanded and, naturally, so too has the amount of data necessary for good performance. BERT, an early AI foundation model released in 2018, had just 354 million trainable parameters, while modern models like GPT-4o and Claude 3.5 have up to trillions of parameters.
Data Network Effects
From the AI developer’s perspective, then, more data is a good thing. But antitrust and competition regulators and enforcers in the U.S. and Europe are increasingly concerned that, like the oil barons of old, a small number of big tech firms with access to vast amounts of consumer data—including Amazon, Apple, Microsoft, Meta/Facebook and Alphabet/Google—can gain an unassailable advantage in the development and deployment of new AI technologies.
These firms typically can’t use all their user data for AI model training purposes due to contractual or legal restrictions. Regulators nonetheless theorize that advantages conferred by user data they can use are enhanced through “data network effects,” whereby access to large amounts of existing data creates a feedback loop for accumulating even more data. For instance, people are drawn to Facebook and Instagram rather than newer or smaller social media platforms because a larger proportion of their friends, relatives and those they may want to interact with are already on these larger, established platforms.
Data network effects also arise because “the more that [a] platform learns from the data it collects on users, the more valuable the platform becomes to each user.” This could make the large platform’s initial advantage virtually impregnable for smaller rivals or those that fall behind. Since feeding “training data” into machine learning models is essential for both their initial development and fine-tuning them for more specific applications, regulators worry that big tech firms and platforms could use their control over data to inhibit competition and innovation in AI by denying rivals access to this essential input, or by coercing them into paying exorbitant prices for it.
A related concern is that incumbent platforms with access to large volumes of data could leverage this advantage to enter and dominate other markets, thereby deterring or inhibiting competition from rivals. For instance, the Department of Justice notes that “[g]eneral search services, search advertising, and general search text advertising require complex algorithms that are constantly learning which organic results and ads best respond to user queries [and] the volume, variety, and velocity of data accelerates the automated learning of search and search advertising algorithms.” Google could thus use data from its market-leading search engine and search advertising services to gain an advantage in product design by better ascertaining the preferences of the consumer base. It would also have an advantage in refining algorithms tailored for other services.
These seemingly commonsensical reasons for regulators’ concerns about “big data,” however, are not fully borne out by market realities. Indeed, an examination of evolving market dynamics suggests that regulators would be well advised to proceed cautiously.
Why ‘Big Data’ Isn’t Everything
Regulators are right that data are crucial for AI development. An AI model is fed data by software engineers or real-time users to refine its inference-making process. Each successive data input allows the model to adjust its predictions to reach a more desired or accurate output. For example, image generators like Midjourney were trained with data inputs including different human-made works of art, thereby allowing them better to produce art that approximates what a human would produce. Similarly, an AI intended to recognize human faces would need to be trained on inputs that include a range of human faces to capture the diversity and variety of features represented in the population.
But what matters isn’t the volume of data alone. Its variety, scope and appropriateness for the AI model’s end goal is just as important, if not more so. For example, the facial recognition model is going to be less accurate and fit for its purpose in a society with a racially diverse population if it’s trained on an input of 1,000 Caucasian faces instead of 500 faces that capture a range of races.
Similarly, an overbroad set of training data can undermine an AI model’s accuracy and ability to achieve its intended outcome. An AI model trained to produce realistic pictures of oranges may produce inaccurate or distorted pictures if its training data include images that contain both oranges and apples. Similarly, Chat-GPT may produce inaccurate answers to research questions if its training data include articles containing misinformation or contradictory information and claims.
Even when there is a large amount of appropriate or representative data, the value of additional inputs into the dataset reduces after a certain point. For instance, a facial recognition model trained on a population-representative sample of 1,000,000 faces may not receive a significant accuracy boost if trained on an additional representative sample of 100,000 faces—its functionality and fitness for its intended purpose would remain nearly the same. And a competing AI facial recognition developer may fail to attract clients and customers away from the other if its only comparative advantage is that it trained its model on a larger representative dataset.
In short, having enough of the right kind of data matters more than simply having more data. Once two (or more) competitors have enough data, innovations in other areas—such as user interface and experience, algorithmic coding and functionality, machine learning/training methods, processing speed, etc.—play a bigger role in positively distinguishing their products or giving one an advantage over the others. Competitors might make better use of the data through superior training techniques or superior data cleaning and categorization, which enhances the efficiency of the data and makes the model more accurate by improving the effectiveness of the machine learning process.
Market Reality
Moreover, regulators’ worry that a few large firms will amass all the data and prevent competition has so far proven unfounded. Incumbent big tech firms such as Google and Meta have thus far failed to outcompete burgeoning startups like OpenAI’s Chat-GPT and Midjourney. This is true despite Google’s and Meta’s ready access to massive volumes of user data and search queries, and despite investing billions of dollars and countless hours into producing their own chatbots and image generators. Six out of every 10 attempts to access AI tools online are estimated to be ChatGPT queries, with OpenAI’s chatbot being nine times as popular as Google’s Bard. ChatGPT has achieved its success using mostly public web data for training. Similarly, in the image generation space, despite having access to a far superior database of images and videos through platforms such as YouTube and Facebook, Google and Meta have also failed to attract as many users as market leaders Midjourney, Dall-E and Stable Diffusion.
Having enough of the right kind of data might be an achievable bar for far more firms than many think, especially given the thriving market of companies that are either curating and offering datasets for free, or selling or licensing access to proprietary datasets on commercial terms. There is currently a thriving market of these datasets: Even tech giants like Google and Meta have released their models on an open-source basis, allowing developers to enter the market cost-free.
Firms have also responded to data scarcity concerns through a thriving industry of secondary data providers or “data warehouses,” which are currently entering the market in droves. Additionally, technological innovation has allowed for “synthetic data”—artificially created datasets that replicate the patterns and parameter correlations of an existing representative dataset, thereby allowing for AI models and applications to be trained on less data while raising fewer user data privacy concerns. Although synthetic data has its limitations, early studies find that models trained on it can deliver equal performance to those utilizing real datasets, indicating that this evolving technology holds substantial promise.
In any case, data access can never trump the role and importance of a superior and innovative algorithm or application. Leading AI model developers are focused less on accumulating data and more on developing the mathematical and logical reasoning capabilities of their models to eventually replicate the abstract insights and creativity that the human mind is capable of. And there is certainly far greater potential for competitors in this space to distinguish themselves from one another in their attempts to achieve this functionality than in their ability to gather data or even their choice of dataset.
Indeed, the incentive even for tech giants like Google and Meta to make their models or data freely or easily available for license might be to increase the odds that a talented programmer, team or firm can make the best use of it through innovations and improvements. Mark Zuckerberg recently clarified as much by claiming this idea as grounds for Meta’s decision to release its LLaMA models on an open-source basis.
Zuckerberg also notes other incentives to making LLaMA models open-source, including expectations of more rapid progress and uptake of the model; alleviation of user concerns regarding data privacy since open ecosystems (unlike many closed ones) can be run from any server of the user’s choice and don’t tie users to the model owner’s servers; and a greater likelihood that Meta’s model will become a standard across more industries and applications due to the rapid refinement and ease of access afforded to developers through open-source access.
As summed up by the International Center for Law and Economics, “data may often confer marginal benefits [but] there is little evidence that these benefits are ultimately decisive.”
Data Network Effects Are Overrated
Antitrust regulators are worried about data network effects giving an unfair advantage to existing large AI developers. Empirical reviews of literature and studies on the magnitude of data network effects, however, have found them to be limited and greatly variable in their impact on a firm’s incumbency advantage.
MIT management science professor Catherine Tucker finds three major flaws in arguments that incumbent tech platforms, such as Meta and Google, have major and increasing network advantages due to the size and scope of their existing user base. First, digital platforms have less of an incumbency advantage than hardware technology firms, as it’s relatively easy to switch digital services to alternative competitors, and lower barriers to enter the market with a competing digital service. Second, this ease of entry and user accumulation generally connotes greater instability in attracting, retaining and/or growing a user base. And third, personalization and individualization play a greater role in attracting and retaining users on digital platforms than on hardware-based or nondigital ones. This increases the potential either for new competitors to attract users from incumbent platforms/services or for these users to use multiple “competitor” platforms and services.
For instance, social media users may be more likely to spend time on or use platforms on which their local community interacts than those with a much more expansive global user pool. And younger users may prefer platforms that are less likely to be frequented by their parents or by older users—a factor that has played a role in the increasing popularity of TikTok and Instagram relative to Facebook.
It would thus be less likely than regulators assume for a tech giant with a massive user data pool to monopolize the market for user data to a degree that would allow them to hurt competition. Indeed, such platforms can gain a competitive advantage by making this data available to innovators and developers who can improve or add to the platform’s services, such as by augmenting the platform’s AI features.
Interoperability Mandates Could Do More Harm Than Good
Regulators have also proposed data interoperability and portability mandates as a way to prevent just a few “big tech” firms from gaining an AI monopoly. These mandates are rules that would require platforms that hold vast amounts of proprietary data to share it with competitors, either freely or in return for limited compensation. Data portability also allows tech platform users to freely shift their data between platforms, thus making it easier for them to switch from one platform to another.
But digital platforms and AI firms already exchange information and data through voluntary arrangements, ostensibly for mutual benefit. Forcing firms to share datasets they’ve painstakingly gathered with competitors for free or at artificially low cost greatly reduces the incentive to gather that data in the first place. If firms can’t realize a commercial return on their data-gathering or platform-development investments, then they may opt for alternative projects that could be more profitable. The firms that are the intended beneficiaries of these mandates would not only miss out on free or low-cost data, but would also not be able to enter into commercial partnerships to acquire it. Thus, competition would be reduced below the current status quo.
Research has also found that even though interfirm data sharing can benefit consumers by allowing smaller firms to compete more effectively, it can harm consumers in cases where data is shared between two relatively evenly matched competitors. In this case, data sharing reduces the incentive for these competitors to compete aggressively to attract each other’s customers (and thus their user data).
Portability mandates also raise user privacy and cybersecurity concerns, especially around personal information including financial, identity or health information. Cyberattacks have become increasingly common, and even tech giants and government agencies with far greater resources to safeguard their servers (and generally higher standards) than smaller firms have been compromised.
With great data come great responsibility. This is especially true as state, national and international bodies propose or pass data privacy legislation, with firms scrambling to recruit technical compliance staff and boost their resources to comply with the law. Portability mandates may force firms that lack these resources to hold data they aren’t prepared to, thus forcing them to either exit the market or incur cost burdens that inhibit their ability to compete—the opposite of what competition regulators ought to want.
Commercial Partnerships and Acquisitions
Regulators, law enforcers and policymakers (and even judges) often ignore the possibility that voluntary interactions among firms or future technological progress could overcome the problems or barriers they seek to avert by fiat. Indeed, they often ignore or downplay the role of even existing arrangements between parties seeking mutual benefit. In the AI space, this could hold true for the very arrangements and mergers between larger and smaller tech firms that have attracted scrutiny from competition enforcers who are concerned that these arrangements will facilitate anticompetitive behavior.
Partnerships between large tech firms and startups can bring together their comparative advantages. Larger firms provide the resources and capital necessary to expeditiously roll out new technologies and navigate the sometimes complex or uncertain legal and regulatory systems around them, while smaller startups bring to bear new innovations. In the case of data-driven AI, larger firms contribute a range of advantages, including monetary investment, platforms for deployment, access to vast databases of users and vital resources such as cloud storage, powerful computing, cutting-edge hardware and long-standing expertise in bringing products from conception to market.
Meanwhile, smaller firms often offer larger firms the innovative technologies necessary to give the latter an edge over its competitors. Additionally, the possibility of getting a substantial investment from a large firm, or even being “bought out,” provides an incentive for talented tech professionals to take a risk and become startup entrepreneurs, a move that usually entails giving up secure, lucrative employment in existing firms.
Smaller firms may also prefer or benefit more from partnerships or mergers with larger firms than with other smaller ones, since the larger firms have more money and are therefore more willing to risk it on unproven technologies. So prohibiting or limiting acquisitions and commercial partnerships between larger and smaller firms can actually reduce competition by discouraging startup entrepreneurship. This, in turn, prevents troves of data held by incumbent firms from being put to their most effective use.
Current market realities, technological progress and the very nature of data’s role in AI technologies make it unlikely that access to vast troves of data gives a substantial enough advantage to incumbent tech platforms for them to reduce competition and innovation. Conversely, competition agencies’ proposed solutions for this speculative problem, including data transfer mandates and scrutiny of commercial partnerships that could bring improved technologies to the market, are likely to do more harm than good for consumers, competition and innovation. When it comes to AI, big data isn’t everything.