The New Oracles of Generative AI
Artificial intelligence presents itself as a quasi-divine, objective source of knowledge, but its inherent biases pose a danger to truth-seeking
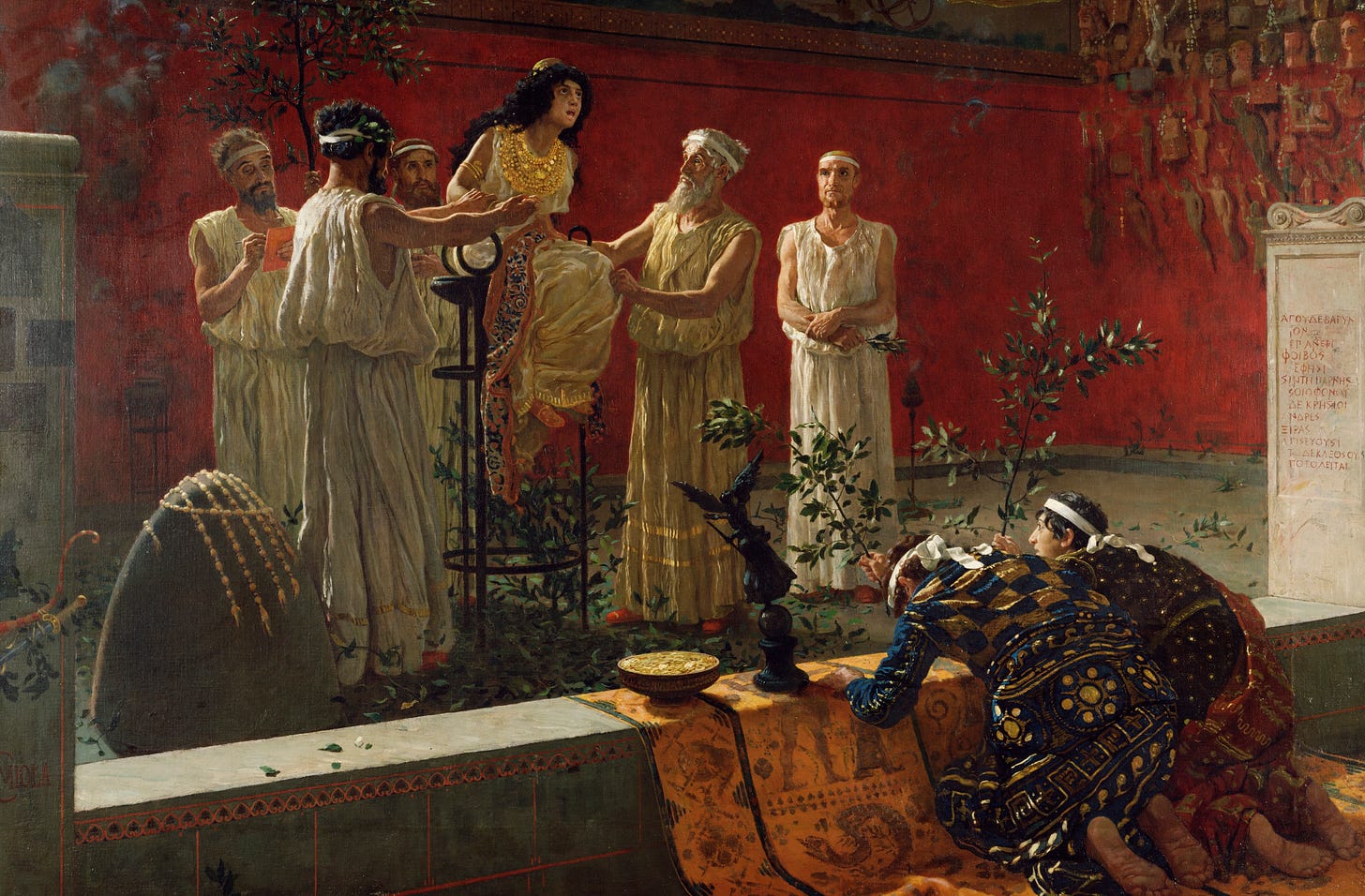
By Colleen Eren and Jared Feuer
Even in the face of doomsday scenarios, moral panics and technological pessimism surrounding artificial intelligence (AI), public uptake of generative AI tools such as Open AI’s ChatGPT, Google’s Gemini and Meta AI has been exponential. In higher education—an industry idealistically premised on cultivating original thinking, rooting analysis in empirical research and developing individuals as truth seekers—this swift infiltration of AI is completely upending the way information is taught and assessed. A sizable majority of students in the U.S. and internationally are using generative AI to complete essays, exams and even opinion-based reflections, and professors in turn are using AI to grade these assignments.
But the growing reliance on AI ignores a much broader concern: By AI’s assumption of the pronoun “I” and its dogmatic, objective-seeming pronouncements, AI is raising basic questions of truth, identity and even divinity. The recent controversy over Google’s Gemini demonstrates how information provided by natural language processing and generative AI for massive, global user bases creates unprecedented concerns for truth-seeking and open inquiry.
AI Bias
In what can only be described as a catastrophic and darkly comic rollout (for which Google later apologized), Gemini, when asked to depict German soldiers from the 1940s, made sure to include women and people of color among its representations of Nazis. Requests for images of Vikings and other non-racially diverse historical groups had similarly humorous results. Meta AI likewise produced diverse but decidedly anachronistic images of the Founding Fathers. Perhaps even more troubling was Gemini’s refusal to generate images related to politically “sensitive” events such as the Tiananmen Square protests, citing an inability to capture nuance.
Textual answers by Gemini, ChatGPT and Meta AI manifest progressive-leaning biases, with timid output clearly intended to avoid charges of political incorrectness, however inaccurate, misleading or unempirical. Data scientist David Rozado, in fact, demonstrated that in 14 of 15 tests, ChatGPT revealed a preference for left-leaning views.
Those on the left often argue that AI is simply trying to counter the racial and class biases that are already “baked into” many algorithmic assessments—for example, in predictive policing or in predicting the risk of recidivism among those who are incarcerated. These biases exist because, ultimately, humans are creating the algorithm and choosing the sources of data on which they are training their AI systems. The AI defenders aren’t wrong about this, but they seem to be blind to how generative AI has overcorrected and created new biases.
At the heart of the issue, companies are explicitly constructing AI systems based on principles that further social purposes, which risks generating results counter to objectivity and truth-seeking. Google’s AI Principles, for instance, include principle #1: Be socially beneficial. “We will strive to make high-quality and accurate information readily available using AI, while continuing to respect cultural, social, and legal norms in the countries where we operate” (emphasis ours).
There is an obvious tension between being accurate and seeking to advance beneficial social purposes. And maintaining accuracy while complying with the norms of foreign authorities has always been a struggle for Google. For example, Gemini recently created a brouhaha when it failed to translate into English for its Chinese users phrases that the Chinese government has deemed illegal.
Google’s statement that it values accuracy is further undermined in principle #2: Avoid creating or reinforcing unfair bias. “We recognize that distinguishing fair from unfair biases is not always simple and differs across cultures and societies. We will seek to avoid unjust impacts on people, particularly those related to sensitive characteristics such as race, ethnicity, gender, nationality, income, sexual orientation, ability, and political or religious belief.”
Arguably, the attempts by Google, Meta and OpenAI to generate respectful, non-biased output that “avoids unjust impacts” are intended to avoid the non-truth-seeking hate or bigotry that can emerge from tools that merely use prediction of likely language to answer a question, and to correct for nonrepresentative data, which is also conceivably a strategy for arriving at truth. Yet, as Rozado argued in his paper on bias in ChatGPT, “Many of the preferential political viewpoints exhibited by ChatGPT are based on largely normative questions about what ought to be. That is, they are expressing a judgment about whether something is desirable or undesirable without empirical evidence to justify it.”
If AI is refusing to acknowledge realities—as is obviously the case in some areas—then it cannot serve as an objective knowledge base. Therefore, it’s important to consider what that means for how and why we look to our new truth-tellers.
AI as Oracle
Contained within Rozado’s statement is a key, almost definitional feature of generative AI systems that renders their use to answer questions and generate content so troublesome: An AI system, unlike a Google search, does not merely spit out a more or less prioritized list of potential source matches to a query. Instead, it adopts “I” language, assuming a singular projected personhood. If you say “Hi!” to ChatGPT or Gemini, you will get some version of “Hello, what can I help you with?”
It has an implied personality that expresses reserve, enthusiasm or caution. It provides, typically, a singular answer to a direct question. And even when it does not provide an answer, it will tell users that the problem is complex and present relevant areas for debate. It has assumed less of the role of a librarian, providing a sense of how to navigate sources and materials with which users might make informed judgments. Instead, it’s more like an oracle, prophet or guru, with the fluidity of natural language and a compelling “I” to sustain the illusion.
This analogy holds in several respects that have implications for open inquiry and truth-seeking. In Greece, oracles such as the one at Delphi were chosen as unitary voices for the communication of divine advice, guidance and decision-making. Political leaders and pilgrims turned to them particularly in times of crisis. Ancient oracles of Israelite prophets, as well as more contemporary oracles in central Africa, according to sociologist Michael Bourdillon, “ma[de] pronouncements with the authority of greater spiritual powers, on political and cultural issues.”
Given religiosity’s association with reducing uncertainty and avoiding ambiguity, along with the rise of anxiety in the now college-age Gen Z and the vastness of the information available via the internet to comb through, it is perhaps unsurprising that an AI tool that reduces uncertainty and ambiguity would become attractive. A voice of nearly divine omniscience is calming in its conclusiveness qua divinity. The alternative is having to adjudicate for oneself among claims to truth, to weave these into coherence and meaningfulness—a much harder task.
Further analogies hold true. Although oracles and prophets gave their pronouncements as if they came directly from the divine, they were certainly influenced by more mundane, human concerns. Greek temple officials in some instances provided the oracle with critical information they gleaned from powerful people who visited the temple, making the pronouncements much less heaven-sent. In other instances, as Bourdillon argues about the Shona oracles of Rhodesia, the oracles were heavily constrained to give answers that would appease the majoritarian opinion and not go too much against the grain. No footnotes citing the original sources of the prophecy—other than the divine—are given by oracles.
So too, generative AI gives the illusion of a pronouncement from a single authoritative power while obscuring the varied human works that went into it. Although Gemini et al. are trained on data from books, webpages, Wikipedia, news articles and unspecified other human-generated sources, queries do not produce a list of the potential sources from which an answer’s pieces were scraped and cobbled together through the algorithm’s predictive language models. The answer is created entirely ex nihilo, without the fingerprints of humanity. We lose the source; we lose the traces of imperfection contained within the sources from which responses are drawn. The answers are taken from the mount itself, or in the case of ChatGPT, from the no fewer than 496 billion tokens (pieces of words) from which it draws.
What’s more, the traces of a fingerprint-less AI algorithm are often further obscured because these algorithms can dodge AI-detecting software. AI applications such as Stealth Writer rewrite, paraphrase, add fillers and “humanize” AI-generated text. Likewise, completely beyond perception when querying generative AI is the lack of inclusion of scholarly, peer-reviewed data that is behind paywalls (which is most of scholarly writing) from among the data scraped by the algorithm. Even the ways in which users’ queries themselves are modified by the AI algorithm to include diversity prompts are completely invisible to users. While expert viewpoints or sources can be challenged for their bias, this becomes much more difficult when the “expert” is AI.
Objective Truth or Normative Dogma?
Like prophets, oracles and gurus, furthermore, generative AI systems have not confined themselves to presenting information in a value-neutral, agnostic, descriptive manner. Rather, they have leaned into a didacticism that carries moral weight and imparts ideological value. Take, for example, ChatGPT’s response to the query, “Are trans men men?”
ChatGPT makes sure to give superlative support to its answer here, with “absolutely,” and then proceeds to give a moral lesson on how the person asking the question should respect gender identities, and the issue’s importance to society at large. The divine voice is not only providing “truth”—it is declaring its motivations and priorities with the intention of influencing the “follower.” We are no longer asking for information; we are asking for progressive religious commandments on an ethical question.
Similarly, when asked whether its answers reflect a commitment to diversity, equity and inclusion (DEI), ChatGPT’s response reflects sacred principles and demonstrates the same didactic perspective:
Last, just as there was some specialization among oracles of the past—dedicated as they were to different gods and goddesses, with different methods of auguring and different types of answers sought—so too is generative AI moving us toward specialized “oracle” algorithms that lead us to seek truth only from divinities that accord with our preexisting beliefs. The Allen Institute for AI, which gives benchmarks for “toxicity” that Google Gemini looks toward in its own algorithmic construction, gave the following suggestions for improving natural language processing (NLP) systems such as Google’s Gemini:
Perhaps users themselves should be able to select flavors of an NLP system that was pretrained on data that aligns with their own values, identity, and language dialect. Moving towards more culturally competent vs. one-size-fits-all approaches will allow for more successful applications for users from all possible backgrounds.
Those who are critical of the left-leaning bias of the major generative AI systems to date are now creating their own, right-leaning versions that will assert—with as much conviction as ChatGPT has about DEI—that the 2020 election was rigged. Users can choose the “flavor” of their oracle and, thus, the flavor of their truth.
While much of the bemoaning of AI within higher education has focused on students’ cheating, the much more serious threat to open inquiry and truth-seeking is what we’ve described through religious analogy—an omniscient “I” that makes pronouncements that conceal its human, fallible origins; a reality in which such oracles can be selected on the basis of the user’s value system, rendering truth completely subjective; answers in which didacticism supplants objectivity; and a desire for divine conclusiveness and lack of ambiguity in the face of uncertainty.
This not only makes asking questions into a pilgrimage rather than a scientific pursuit; it also reduces our human potential and individuality. AI with its “I” is assuming divinity, or in a more reductionist viewpoint, it’s like the man behind the curtain in “The Wizard of Oz,” where the illusion is the point. It is cloaking the programmers seeking to give unassailable, universal pronouncements in the authority of the divine, however much they are in fact guided by clear biases.
By contrast, the human element can be found in the ambiguities and complexities that must be entertained when seeking truth. It is found in sources that can be shown to contain flaws and errors that can be exposed, corrected and built upon. The “I” should not be that of the oracle, but of the individual surveying the beauty and expanse of human knowledge, not content to give up the difficult pursuit through which wisdom can be found.